Failure Detection in Induction Motors Using Non-supervised Machine Learning Algorithms
Abstract:
Induction motors are widely used around the world in many industrial and commercial applications. Early detection of faults in these devices is important to avoid service disruption and increase their useful life. Thus, many non-invasive schemes have been proposed to detect failures in induction motors using machine learning techniques mainly. Many of these machine learning algorithms are based on supervised training, requiring examples of motors with and without failures. Collecting training data with positive and negative examples is certainly a time consuming and costly task at the field. Hence, this work proposes to use non-supervised training algorithms to detect failures in induction motors using exclusively measurements in healthy motors. Specifically, two non-supervised learning algorithms, namely k-means and self-organizing maps, are employed to detect broken bars and faulty bearings. Final results are compared to supervised training algorithms, showing that non-supervised learning algorithms are also able to detect motor failures with high accuracy, and probing that robust models can be built using only healthy-motor data.
Año de publicación:
2021
Keywords:
- Induction Motors
- Machine learning algorithms
- Failure detection
- Non-supervised learning
Fuente:
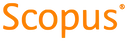
Tipo de documento:
Conference Object
Estado:
Acceso restringido
Áreas de conocimiento:
- Software
- Aprendizaje automático
Áreas temáticas:
- Física aplicada
- Métodos informáticos especiales