Fall detection system for elderly people using IoT and ensemble machine learning algorithm
Abstract:
Falls represent a major public health risk worldwide for the elderly people. A fall not assisted in time can cause functional impairment in an elderly and a significant decrease in his mobility, independence, and life quality. In this sense, we propose IoTE-Fall system, an intelligent system for detecting falls of elderly people in indoor environments that takes advantages of the Internet of Thing and the ensemble machine learning algorithm. IoTE-Fall system employs a 3D-axis accelerometer embedded into a 6LowPAN wearable device capable of capturing in real time the data of the movements of elderly volunteers. To provide high efficiency in fall detection, in this paper, four machine learning algorithms (classifiers): decision trees, ensemble, logistic regression, and Deepnets are evaluated in terms of AUC ROC, training time and testing time. The acceleration readings are processed and analyzed at the edge of the network using an ensemble-based pbkp_redictor model that is identified as the most suitable pbkp_redictor for fall detection. The experiment results from collection data, interoperability services, data processing, data analysis, alert emergency service, and cloud services show that our system achieves accuracy, precision, sensitivity, and specificity above 94%.
Año de publicación:
2019
Keywords:
- random forest
- Accelerometer sensor
- Fall detection
- internet of things
- Ensemble learning algorithm
- IoT gateway
- Elderly people
- 6LoWPAN
Fuente:
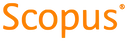
Tipo de documento:
Article
Estado:
Acceso restringido
Áreas de conocimiento:
- Aprendizaje automático
- Ciencias de la computación
Áreas temáticas de Dewey:
- Ciencias de la computación