Fast and robust ℓ1-averaging-based pose estimation for driving scenarios
Abstract:
Robust visual pose estimation is at the core of many computer vision applications, being fundamental for Visual SLAM and Visual Odometry problems. During the last decades, many approaches have been proposed to solve these problems, being RANSAC one of the most accepted and used. However, with the arrival of new challenges, such as large driving scenarios for autonomous vehicles, along with the improvements in the data gathering frameworks, new issues must be considered. One of these issues is the capability of a technique to deal with very large amounts of data while meeting the realtime constraint. With this purpose in mind, we present a novel technique for the problem of robust camera-pose estimation that is more suitable for dealing with large amount of data, which additionally, helps improving the results. The method is based on a combination of a very fast coarse-evaluation function and a robust ℓ1-averaging procedure. Such scheme leads to high-quality results while taking considerably less time than RANSAC. Experimental results on the challenging KITTI Vision Benchmark Suite are provided, showing the validity of the proposed approach.
Año de publicación:
2013
Keywords:
Fuente:
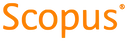
Tipo de documento:
Conference Object
Estado:
Acceso abierto
Áreas de conocimiento:
- Visión por computadora
Áreas temáticas:
- Métodos informáticos especiales