Fast prediction of loadability margins using neural networks to approximate security boundaries of power systems
Abstract:
Determining loadability margins to various security limits is of great importance for the secure operation of a power system, especially in the current deregulated environment. Here, a novel approach is proposed for fast prediction of loadability margins of power systems based on neural networks. Static security boundaries, comprised of static voltage stability limits, oscillatory stability limits and other operating limits such as generator power output limits, are constructed by means of loading the power system until these security limits are reached from a base operating point along various loading directions. Back-propagation neural networks for different contingencies are trained to approximate the security boundaries. A search algorithm is then employed to predict the loadability margins from any stable operating points along arbitrary loading directions through an iterative technique based on the trained neural networks. The simulation results for the IEEE two-area benchmark system and the IEEE 50-machine test system demonstrate the effectiveness of the proposed method for on-line prediction of loadability margins. © The Institution of Engineering and Technology 2007.
Año de publicación:
2007
Keywords:
Fuente:
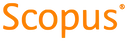
Tipo de documento:
Article
Estado:
Acceso restringido
Áreas de conocimiento:
- Potencia eléctrica
- Red neuronal artificial
- Energía
Áreas temáticas de Dewey:
- Física aplicada
- Métodos informáticos especiales

Objetivos de Desarrollo Sostenible:
- ODS 7: Energía asequible y no contaminante
- ODS 8: Trabajo decente y crecimiento económico
- ODS 9: Industria, innovación e infraestructura
