Fault Diagnosing for Electric Motors Based in Noise Data Classification Using Deep Learning
Abstract:
Timely detection of potential engine failures is essential for preventive maintenance of equipment. This avoids damages to machines, potential security risks and reduce high costs involved in repairing or replacing them. The audio emitted by machines during operation is an indicator of a potential engine failure. Audio-based fault classification and detection is a research area dominated by solutions based on AutoEncoder Neural Networks. In this work, an end-to-end approach is proposed for classifying electric motor noise. The methodology includes a pre-processing phase to transform the audio time series into a matrix representation of spectrogram images, capturing time and frequency domain data, then classifying them using an Ensemble of Convolutional Neural Network models. To evaluate the performance a set of metrics have been measured and compared, reaching a performance accuracy above 97% and AUC above 99%. Therefore, acoustic registry of electric motors could also be a powerful source of useful data to include in pbkp_redictive maintenance data analysis.
Año de publicación:
2022
Keywords:
- Engine Failures
- Audio Anomaly Classification
- Spectrogram Analysis
- deep learning
Fuente:
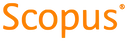
Tipo de documento:
Conference Object
Estado:
Acceso restringido
Áreas de conocimiento:
- Software
- Aprendizaje profundo
Áreas temáticas de Dewey:
- Física aplicada
- Instrumentos de precisión y otros dispositivos
- Métodos informáticos especiales