Fault Diagnosis for 3D Printers Using Suboptimal Networked Deep Learning
Abstract:
For reliable and cost-saving diagnosis of 3D printer faults, a customer-goods level attitude sensor is employed to collect healthy condition data of the printer. To make up for the inferior precision of the low-cost hardware, a suboptimal networked deep learning (SNDL) method is developed. In addition to conventional deep learning architecture composed of pre-training and fine-tuning, there are two new merits for SNDL. A pre-classifying strategy is first proposed to adaptively determine the suboptimal network structure. A fine-classifying approach is on the other hand adopted for further improving fault classification performance. In the experiments, the attitude sensor is installed on the end of the transmission chain (i.e., the printing head of the delta 3D printer). Motion signals collected from all channels of the sensor are used as the input vector of the suboptimal networks, which are developed by deep Boltzmann machines for the big data-driven fault diagnosis. The addressed SNDL method is compared with peer methods. The results show that the present SNDL is capable of effectively diagnosing transmission faults for the 3D printer.
Año de publicación:
2019
Keywords:
- Suboptimal network
- BIG DATA
- 3D Printing
- deep learning
- Fault diagnosis
Fuente:
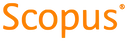
Tipo de documento:
Article
Estado:
Acceso abierto
Áreas de conocimiento:
- Aprendizaje automático
- Ciencias de la computación
Áreas temáticas:
- Métodos informáticos especiales
- Física aplicada