Fault diagnosis in reciprocating compressor bearings: an approach using LAMDA applied on current signals
Abstract:
Condition monitoring is one of the most important activities to implement pbkp_redictive maintenance in industrial processes and perform fault diagnosis. Vibration is the most used signal for this purpose, however current signals arise as a non-intrusive alternative to condition monitoring. On the other hand, data driven approaches becomes as a way to develop fault classifiers by using Machine Learning. This paper proposes the development of a fault classifier for diagnosing failures in the bearings of a reciprocating compressor by using the current signals measured from the induction machine that power the mechanical device. The proposal applies cluster validity assessment for feature selection, and a LAMDA-based model for classification. Results show that this proposal can diagnose three failure modes with a precision over 90%.
Año de publicación:
2022
Keywords:
- Cluster validity index
- fuzzy similarity
- reciprocating compressors
- Anova
- Fault diagnosis
- feature selection
Fuente:
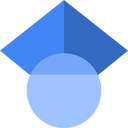
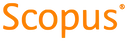
Tipo de documento:
Conference Object
Estado:
Acceso abierto
Áreas de conocimiento:
- Ingeniería mecánica
- Aprendizaje automático
Áreas temáticas:
- Física aplicada