Feature selection based on reinforcement learning for object recognition
Abstract:
This paper presents a novel method that allows learning the best feature that describes a given image. It is intended to be used in object recognition. The proposed approach is based on the use of a Reinforcement Learning procedure that selects the best descriptor for every image from a given set. In order to do this, we introduce a new architecture joining a Reinforcement Learning technique with a Visual Object Recognition framework. Furthermore, for the Reinforcement Learning, a new convergence and a new strategy for the exploration-exploitation trade-off is proposed. Comparisons show that the performance of the proposed method improves by about 6.67% with respect to a scheme based on a single feature descriptor. Improvements in the convergence speed have been also obtained using the proposed exploration-exploitation trade-off.
Año de publicación:
2012
Keywords:
- Object recognition
- Visual feature descriptors
- Q-Learning
- reinforcement learning
Fuente:
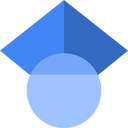
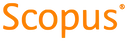
Tipo de documento:
Conference Object
Estado:
Acceso restringido
Áreas de conocimiento:
- Aprendizaje automático
- Ciencias de la computación
- Ciencias de la computación
Áreas temáticas:
- Métodos informáticos especiales