Feature selection in gait classification using geometric PSO assisted by SVM
Abstract:
Gait recognition is used to identify individuals by the way they walk. Recent research in automated human gait recognition has mainly focused on developing robust features representations and matching algorithms. To our best knowledge, feature selection is rarely addressed in gait classification problems. In this paper, we evaluate the performance of a particle swarm optimization (PSO) algorithm assisted by a support vector machine (SVM) for feature selection in gait classification. In this way, while PSO generates trial feature subsets, SVM estimates their fitness value during the search process. The resulting subset is evaluated by means of a SVM classifier to obtain the fitness value (correct classification rate) of such particle. The performance of the proposed approach is evaluated by using the well-known Southampton covariate database (SOTON). Our experimental results indicate that our proposed approach is able to achieve highly competitive results with respect to state-of-the-art approaches adopted in our comparative study.
Año de publicación:
2015
Keywords:
- Gait classification
- SUPPORT VECTOR MACHINES
- Particle Swarm Optimization
- feature selection
Fuente:
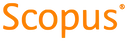
Tipo de documento:
Conference Object
Estado:
Acceso restringido
Áreas de conocimiento:
- Aprendizaje automático
- Ciencias de la computación
Áreas temáticas:
- Ciencias de la computación