Fine-tuning a pre-trained Convolutional Neural Network Model to translate American Sign Language in Real-time
Abstract:
In this paper, we present a real-time American Sign Language (ASL) hand gesture recognizer based on an artificial intelligence execution, instead of the classical and outdated image processing modalities. Our approach uses a Convolutional Neural Network (CNN) to train a dataset of hundreds of instances from the ASL alphabet, extracting the features from each and every pixel and constructing an accurate translator based on predictions. This approach employs an atypical trade-off for a translator, where a superior precision and speed at the inference phase compensates for the computational expense at the early training. Furthermore, and to the best of our knowledge, the accuracy obtained by using the proposed deep learning technique, surpass the accuracy obtained using non-machine learning practices. The performance obtained by the proposed algorithm has also been compared with existing literature, showing that the suggested methodology outperformed the accuracy of its analogous counterparts.
Año de publicación:
2019
Keywords:
- real-time
- image classification
- Convolutional neural network
- Transfer learning
- Sign Language
- Artificial Intelligence
Fuente:
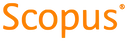
Tipo de documento:
Conference Object
Estado:
Acceso restringido
Áreas de conocimiento:
- Aprendizaje automático
- Ciencias de la computación
- Ciencias de la computación
Áreas temáticas de Dewey:
- Ciencias de la computación
- Métodos informáticos especiales
- Funcionamiento de bibliotecas y archivos

Objetivos de Desarrollo Sostenible:
- ODS 4: Educación de calidad
- ODS 10: Reducción de las desigualdades
- ODS 9: Industria, innovación e infraestructura
