First- and second-order optimality conditions for piecewise smooth objective functions
Abstract:
Any piecewise smooth function that is specified by an evaluation procedure involving smooth elemental functions and piecewise linear functions like min and max can be represented in the so-called abs-normal form. By an extension of algorithmic, or automatic, differentiation, one can then compute certain first- and second-order derivative vectors and matrices that represent a local piecewise linearization and provide additional curvature information. On the basis of these quantities, we characterize local optimality by first- and second-order necessary and sufficient conditions, which generalize the corresponding Kuhn-Tucker-Karush (KKT) theory for smooth problems. The key assumption is the linear independence kink qualification, a generalization of Linear Independence Constraint Qualification (LICQ) familiar from nonlinear optimization. It implies that the objective has locally a so-called vu decomposition and renders everything tractable in terms of matrix factorizations and other simple linear algebra operations. By yielding descent directions, whenever they are violated the new optimality conditions point the way to a superlinearly convergent generalized Quadratic Program solver, which is currently under development. We exemplify the theory on two nonsmooth examples of Nesterov.
Año de publicación:
2016
Keywords:
- Karush–Kuhn–Tucker
- Piecewise linearization
- decomposition
- tangential stationarity
- projected Hessian
- second-order optimality
- normal growth
- abs-normal form
Fuente:
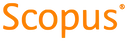
Tipo de documento:
Article
Estado:
Acceso restringido
Áreas de conocimiento:
- Optimización matemática
- Optimización matemática
Áreas temáticas:
- Programación informática, programas, datos, seguridad