Forecast UPC-Level FMCG Demand, Part IV: Statistical Ensemble
Abstract:
Improving forecast accuracy is a major goal of statisticians and forecast practitioners. over the years, many advanced models have been proposed in forecasting studies in various science and engineering domains. It is no exception for the fast-moving-consumer-goods (FMCG) demand forecasting. However, in the literature of FMCG forecasting, there are many contradictory conclusions about the best model, typified by the long-lasting »war» between econometrics and neural networks. This is simply because there is no universal model. Whereas one model may outperform another over one dataset, its performance can be rather limited over other datasets. Hence, when forecasting is required for a new dataset, a forecaster is risking a suboptimal performance, if the model selection is based on other datasets. To minimize that risk, ensemble forecasting is often considered. This paper continues from the previous discussion (see Parts I-III) on UPC-level FMCG demand forecasting. Various statistical ensemble techniques are used to combine forecasts made using a collection of component models. It is found that the risk (measured by the spread of model-led error) of using ensemble is smaller than using a single component model. Furthermore, in a big data environment, automatic ensemble forecasting is preferred over the time-consuming and task-specific model tuning procedure, which is impractical if there are thousands, or even millions, of time series to be forecast.
Año de publicación:
2019
Keywords:
- Forecast combination
- forecasting
- Fast moving consumer goods
- ensemble
Fuente:
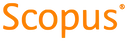
Tipo de documento:
Conference Object
Estado:
Acceso restringido
Áreas de conocimiento:
- Inferencia estadística
- Investigación cuantitativa
Áreas temáticas:
- Probabilidades y matemática aplicada
- Métodos informáticos especiales
- Sistemas