Forecasting Energy Consumption in Residential Department Using Convolutional Neural Networks
Abstract:
During 2017, the construction and operation of buildings worldwide represented more than a third (36%) of the final energy used and 40% of the carbon dioxide emissions. Hence, in the last decade, there has been great interest in analyzing the energy efficiency in buildings from different approaches. In this paper, black-box approaches based on artificial neural networks to predict the energy consumption of a selected residential department building are proposed. The potential of convolutional neural networks (CNN) applied to images and videos is tested in time series as one-dimensional (1D) sequences. CNN models and other combinations with Long Short-Term Memory (LSTM) such as CNN-LSTM and ConvLSTM are proposed to make predictions in two scenarios, i.e., for predicting energy consumption in the next 24 h and 7 days. The results showed that the best model was CNN for the first scenario, and in the second scenario, CNN-LSTM performed better. These models can be very useful in predictive control systems considered in buildings to foresee with great precision the energy consumption behavior in the short, medium, and long term.
Año de publicación:
2021
Keywords:
- Energy efficiency
- cnn
- ConvLSTM
- TIME SERIES
- buildings
- CNN-LSTM
- Pbkp_rediction models
Fuente:
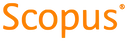
Tipo de documento:
Conference Object
Estado:
Acceso restringido
Áreas de conocimiento:
- Aprendizaje automático
- Energía
- Simulación por computadora
Áreas temáticas de Dewey:
- Métodos informáticos especiales

Objetivos de Desarrollo Sostenible:
- ODS 7: Energía asequible y no contaminante
- ODS 11: Ciudades y comunidades sostenibles
- ODS 9: Industria, innovación e infraestructura
