Forecasting travel times with space partitioning methods
Abstract:
Roads and streets are more and more crowded. For delivery companies that use road transportation, this is a concerning issue as longer times spent on roads mean higher operational costs and less customer satisfaction. Nevertheless, the data captured during operation hours of their vehicles can be leveraged to address such issues. This, however, is not a straightforward task given the possible low number of vehicles covering one route and the complexities introduced by the delivery business nature. The present research work proposes an approach to forecast travel time through the use of probe data from logistic vehicles and simple mathematical models. The delivery operations of five months of a vehicle from the Swiss Post, the national postal service company of Switzerland, were studied in a segment-to-segment manner, following a four-step method. Moreover, the results of the forecasting were evaluated calculating the mean absolute percentage error and mean absolute error metrics. The results obtained indicate that is possible to achieve a considerable forecasting accuracy without the deployment of a large number of vehicles or the implementation of complex algorithms.
Año de publicación:
2020
Keywords:
- transportation
- Smart Logistics
- spatio-temporal data
- geogrid
- Geohash
- Travel Time
Fuente:
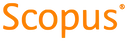
Tipo de documento:
Conference Object
Estado:
Acceso restringido
Áreas de conocimiento:
- Análisis de datos
- Transporte
Áreas temáticas:
- Métodos informáticos especiales
- Otras ramas de la ingeniería
- Transporte