Fully Autonomous Deep Learning RGB-D Vision-based Object Manipulation with an Anthropomorphic Robotic Hand
Abstract:
Fully autonomous object grasping with robotic hands is under active investigation because autonomous vision and motor control is required. Vision allows a robotic hand interact with the environment by estimating the grasping parameters (ie, grasping position and orientation) for manipulation. Motor control generates the motion parameters to reach an object and manipulate (eg, grasping and relocation). In this work, deep learning RGB-D vision is used to detect the object and generate the grasping parameters of position and orientation. An anthropomorphic robotic hand system composed of UR3 robotic arm and qb soft hand is used for motor functions of object grasping and relocation. Our autonomous object manipulation system first detects and locates an object from RGB images using FastRCNN. Then, a partial depth view of the object is generated to estimate the grasping position and orientation of the object. Finally, the robotic hand system is used to grasp and relocate the object. Our autonomous object manipulation system is validated by grasping and relocating a single object of box and ball. For the box, our system achieves 8/10 successful grasping and 7/10 successful relocations, and for the ball 10/10 successful grasping and relocations.
Año de publicación:
2021
Keywords:
Fuente:
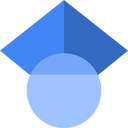
Tipo de documento:
Other
Estado:
Acceso abierto
Áreas de conocimiento:
- Robótica
Áreas temáticas:
- Métodos informáticos especiales