Function approximation with neural networks for obtaining an operating point sufficiently small signal stable in power systems including wind parks
Abstract:
This paper shows a simple approach to obtain an operating point sufficiently small signal stable, using function approximation with neural networks. The idea is to use a neural network to pbkp_redict system's eigenvalues, taking as input data voltage at buses, generated power, reactive load, and the output data are the eigenvalues. Unstable and Poorly damped modes are identified and then these modes will be damped. The system modifies the parameters until reach a stable operating point. In the case of a stable operating point with a poorly damped oscillatory mode, the objective is to increase the damping of that mode. That is, the power system linearization at the operating point is modified. Operator actions such as bkp_redispatch, varying load, varying reactive power (voltage) often modify the operating point to do this; the effect of this is that transients near enough to the operating point will decay more quickly. However, the analysis does not attempt the more difficult study of large signal transients. The existence of a stable operating point is of course necessary for system security, but there is no guarantee that large signal transients will result in operation at that operating point. © 2009 IEEE.
Año de publicación:
2009
Keywords:
Fuente:
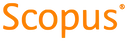
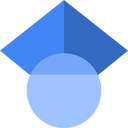
Tipo de documento:
Conference Object
Estado:
Acceso restringido
Áreas de conocimiento:
- Energía
- Aprendizaje automático
Áreas temáticas:
- Física aplicada
- Ciencias de la computación
- Métodos informáticos especiales