Fuzzy case-based-reasoning-based imputation for incomplete data in software engineering repositories
Abstract:
Missing data is a serious issue in software engineering because it can lead to information loss and bias in data analysis. Several imputation techniques have been proposed to deal with both numerical and categorical missing data. However, most of those techniques used is simple reuse techniques originally designed for numerical data, which is a problem when the missing data are related to categorical attributes. This paper aims (a) to propose a new fuzzy case-based reasoning (CBR) imputation technique designed for both numerical and categorical data and (b) to evaluate and compare the performance of the proposed technique with the k-nearest neighbor (KNN) imputation technique in terms of error and accuracy under different missing data percentages and missingness mechanisms in four software engineering data sets. The results suggest that the proposed fuzzy CBR technique outperformed KNN in terms of imputation error and accuracy regardless of the missing data percentage, missingness mechanism, and data set used. Moreover, we found that the missingness mechanism has an important impact on the performance of both techniques. The results are encouraging in the sense that using an imputation technique designed for both categorical and numerical data is better than reusing methods originally designed for numerical data.
Año de publicación:
2020
Keywords:
- categorical data
- Missing data
- empirical software engineering
- accuracy
- imputation
- fuzzy analogy
Fuente:
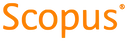
Tipo de documento:
Article
Estado:
Acceso restringido
Áreas de conocimiento:
- Ingeniería de software
- Software
Áreas temáticas de Dewey:
- Ciencias de la computación