Fuzzy classification rules with frvarpso using various methods for obtaining fuzzy sets
Abstract:
Having strategies capable of automatically generating classification rules is highly useful in any decision-making process. In this article, we propose a method that can operate on nominal and numeric attributes to obtain fuzzy classification rules by combining a competitive neural network with an optimization technique based on variable population particle swarms. The fitness function that controls swarm movement uses a voting criterion that weights, in a fuzzy manner, numeric attribute participation. The efficiency and efficacy of this method are strongly conditioned by how membership functions to each of the fuzzy sets are established. In previous works, this was done by partitioning the range of each numeric attribute at equal-length intervals, centering a triangular function with appropriate overlap in each of them. In this case, an improvement to the fuzzy set generation process is proposed using the Fuzzy C-Means methods. The results obtained were compared to those yielded by the previous version using 11 databases from the UCI repository and three databases from the Ecuadorian financial system – one from a cbkp_redit and savings cooperative and two from banks that grant productive and non-productive cbkp_redits as well as microcbkp_redits. The results obtained were satisfactory. At the end of the article, our conclusions are discussed and future research lines are suggested.
Año de publicación:
2020
Keywords:
- Fuzzy C-means
- Index Terms—FRvarPSO (Fuzzy Rules variable Particle Swarm Optimization)
- Classification rules
- fuzzy rules
- Data Mining
Fuente:
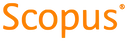
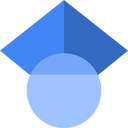
Tipo de documento:
Article
Estado:
Acceso abierto
Áreas de conocimiento:
- Inteligencia artificial
- Lógica difusa
- Ciencias de la computación
Áreas temáticas:
- Métodos informáticos especiales