Fuzzy dynamic parameter adaptation for particle swarm optimization of modular granular neural networks applied to time series pbkp_rediction
Abstract:
In this paper the optimization of Modular Granular Neural Networks (MGNNs) using a Particle Swarm Optimization (PSO) approach is proposed. PSO uses dynamic parameter adaptation based on Fuzzy Logic (FL). The MGNN is applied to time series pbkp_rediction. To perform comparisons with the achieved results using the fuzzy dynamic adaptation of the PSO, tests with non-optimized trainings and runs with a conventional PSO are performed. The fuzzy dynamic parameters of the PSO are c1, c2 and w, as these parameters are essential to have a better PSO performance. The optimization finds optimal parameters of the MGNNs such as: the number of sub modules, number of hidden layers with their number of neurons and goal error. The effectiveness of the proposed method is tested using 800 data points of the Mackey-Glass time series. Tests using 500 and 300 data points for the training phase are performed for …
Año de publicación:
2021
Keywords:
Fuente:
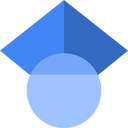
Tipo de documento:
Other
Estado:
Acceso abierto
Áreas de conocimiento:
- Aprendizaje automático
- Algoritmo
- Ciencias de la computación
Áreas temáticas:
- Ciencias de la computación