GSVM: An SVM for handling imbalanced accuracy between classes inbi-classification problems
Abstract:
A new support vector machine, SVM, is introduced, called GSVM, which is specially designed for bi-classification problems where balanced accuracy between classes is the objective. Starting from a standard SVM, the GSVM is obtained from a low-cost post-processing strategy by modifying the initial bias. Thus, the bias for GSVM is calculated by moving the original bias in the SVM to improve the geometric mean between the true positive rate and the true negative rate. The proposed solution neither modifies the original optimization problem for SVM training, nor introduces new hyper-parameters. Experimentation carried out on a high number of databases (23) shows GSVM obtaining the desired balanced accuracy between classes. Furthermore, its performance improves well-known cost-sensitive schemes for SVM, without adding complexity or computational cost. © 2013 Elsevier B.V.
Año de publicación:
2014
Keywords:
- Cost-sensitive schemes
- Imbalanced accuracy
- Imbalanced databases
- classification
- SUPPORT VECTOR MACHINES
Fuente:
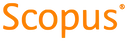
Tipo de documento:
Article
Estado:
Acceso restringido
Áreas de conocimiento:
- Aprendizaje automático
- Algoritmo
Áreas temáticas:
- Métodos informáticos especiales
- Programación informática, programas, datos, seguridad