Gear crack level classification by using KNN and time-domain features from acoustic emission signals under different motor speeds and loads
Abstract:
Diagnosing failures during their initial stage is important to avoid unexpected stops and catastrophic damages, specially for gear boxes that are crucial components in industrial machines. This work addresses the classification of nine levels of crack failure severity in a gearbox. First of all, features are extracted in time domain from signals coming from an acoustic emission (AE) sensor, and then selected by using four different ranking methods. The classification stage uses the k-Nearest Neighbors (KNN) technique. The results indicate that presented levels of severity can be successfully classified with five features extracted from the AE signal for the four ranking methods.
Año de publicación:
2019
Keywords:
Fuente:
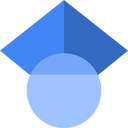
Tipo de documento:
Other
Estado:
Acceso abierto
Áreas de conocimiento:
- Ingeniería mecánica
- Aprendizaje automático
- Ingeniería mecánica
Áreas temáticas:
- Física aplicada
- Métodos informáticos especiales
- Otras ramas de la ingeniería