Gearbox Broken Tooth Severity Classification using EMD of Acoustic Emission Signals
Abstract:
Fault severity classification is a critical task necessary for optimal pbkp_redictive maintenance to reduce costs and avoid catastrophic accidents in the industry. In this research, we propose a methodology for broken tooth severity classification in a gearbox using digital signal processing techniques of acoustic emission signals. The method uses empirical mode decomposition of the signal and extraction of time-domain features from a set of Intrinsic Mode Functions. The extracted features are fed to random forest and linear discriminant analysis models for attaining the classification of nine different severity conditions. The method provides classification accuracies higher than 90% with both machine learning models.
Año de publicación:
2022
Keywords:
- Acoustic Emission
- Broken tooth severity
- empirical mode decomposition
- random forest
- linear discriminant analysis
- gearbox
Fuente:
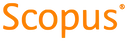
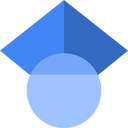
Tipo de documento:
Conference Object
Estado:
Acceso restringido
Áreas de conocimiento:
- Ingeniería mecánica
- Aprendizaje automático
- Ingeniería mecánica
Áreas temáticas:
- Física aplicada