Gender and age classification based on human features to detect illicit activity in suspicious sites
Abstract:
We developed a method to recognize gender and age groups using facial features and upper body proportions. We used scraping images from sites that were classified as suspicious to perform illicit activities with people under the consent age. In these places, the facial features were often blurred, and the classification had to be made with only information of upper body shape and proportions. The proposed method uses Haar-like features for the extraction of characteristics. We obtained a shape description for the face and upper body, and then we extract features and evaluate them according to the spatial relationships and angles between these characteristics. Lastly, we developed SVM algorithms and apply them to each feature to classify classes: male or female and to distinguish people within the group of 14 years old or less. For comparison purposes, we also use Convolutional Neural Networks to categorize the mentioned classes. The attained results were satisfactory enough after five iterations. However, CNN had a much larger execution time than the SVM algorithm. Also, CNN did not perform as well when facial features were not available. Therefore, we considered CNN not suitable for monitoring a large number of sites in the context of our research. Thus, we suggest the use of the procedure with Haar-like features and SVM classifiers.
Año de publicación:
2019
Keywords:
- Age group recognition
- face recognition
- Gender classification
- Upper body proportions recognition
- human trafficking
Fuente:
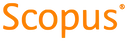
Tipo de documento:
Conference Object
Estado:
Acceso restringido
Áreas de conocimiento:
- Aprendizaje automático
- Ciencias de la computación
Áreas temáticas:
- Otros problemas y servicios sociales
- Interacción social
- Criminología