Generation and reduction of fuzzy sets with PG-means and fuzzy similarity measures
Abstract:
The probabilistic clustering techniques can be applied to generate fuzzy sets in situations where there is little or no information about data. Quite often, they generate a huge number of clusters. These clusters can be interpreted as fuzzy sets in a process of building a fuzzy system. A large number of fuzzy sets introduce noise to the fuzzy system, hence the need to reduce their number. Fuzzy Similarity Measures (FSMs) are widely used for comparison of fuzzy sets. Multiple FSMs have been proposed so far, but identifying a single FSM that is the most suitable for a given task is not always a straightforward process. On many occasions, FSMs are used to reduce a number of fuzzy sets. In this paper, we present the results of analyzing suitability of FSMs to reduce number of fuzzy sets and fuzzy if-then rules for an image segmentation problem. We use a PG-Means algorithm to generate fuzzy sets on both input and output variables. We propose and apply algorithms utilizing FSMs to reduce the number of fuzzy sets and rules. The paper includes a case study investigating the application of the proposed method on two images.
Año de publicación:
2018
Keywords:
- Fuzzy sets reduction
- Fuzzy similarity measures
- PG-means
- fuzzy sets
Fuente:
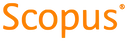
Tipo de documento:
Book Part
Estado:
Acceso restringido
Áreas de conocimiento:
- Inteligencia artificial
- Ciencias de la computación
- Lógica difusa
Áreas temáticas:
- Ciencias de la computación