Genetic algorithm assisted by a SVM for feature selection in gait classification
Abstract:
Feature selection is considered an important step for gait pattern recognition. The task of a gait classifier could be simplified by eliminating redundant and irrelevant attributes for classification. With that, the size of the feature set could be reduced and subsequently a more comprehensible analysis of the extracted patterns could be carried out. In this paper, we present a feature selection method for gait recognition which uses a genetic algorithm (GA) assisted by a support vector machine (SVM). In this way, while the GA obtains a trial feature subset, SVM estimates the fitness value of each new subset generated during the evolutionary process. Our proposed approach is evaluated by using the well-known Southampton covariate database (SOTON). Our experimental study shows the effectiveness of the proposed method when 17% of the undesirable gait features are removed without lose of significant accuracy in the classification process. In order to validate our proposed approach we compare its result with respect to those produced by three state-of-the-art algorithms taken from the specialized literature. Preliminary results indicate that our proposed method not only reduce in an effective way the features of the data model, but also it is able to classify in a proper way the SOTON database.
Año de publicación:
2014
Keywords:
- feature selection
- Gait classification
- genetic algorithm (GA)
- support vector machine (SVM)
Fuente:
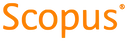
Tipo de documento:
Conference Object
Estado:
Acceso restringido
Áreas de conocimiento:
- Aprendizaje automático
- Algoritmo
Áreas temáticas:
- Ciencias de la computación