Global vs local search on multi-objective NK-landscapes: Contrasting the impact of problem features
Abstract:
Computationally hard multi-objective combinatorial optimization problems are common in practice, and numerous evolutionary multiobjective optimization (EMO) algorithms have been proposed to tackle them. Our aim is to understand which (and how) problem features impact the search performance of such approaches. In this paper, we consider two prototypical dominance-based algorithms: a global EMO strategy using an ergodic variation operator (GSEMO) and a neighborhood-based local search heuristic (PLS). Their respective runtime is estimated on a benchmark of combinatorial problems with tunable ruggedness, objective space dimension, and objective correlation (ρ;MNK-landscapes). In other words, benchmark parameters define classes of instances with increasing empirical problem hardness; we enumerate and characterize the search space of small instances. Our study departs from simple performance comparison to systematically analyze the correlations between runtime and problem features, contrasting their association with search performance within and across instance classes, for both chosen algorithms. A mixed-model approach then allows us to further generalize from the experimental design, supporting a sound assessment of the joint impact of instance features on EMO search performance.
Año de publicación:
2015
Keywords:
Fuente:
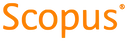
Tipo de documento:
Conference Object
Estado:
Acceso restringido
Áreas de conocimiento:
- Algoritmo
- Optimización matemática
Áreas temáticas:
- Ciencias de la computación