Hammerhead shark detection using regions with convolutional neural networks
Abstract:
Over the years, the illegal catch of sharks in the Pacific Ocean for Asians fin markets has drastically increased, to the point where the Scalloped Hammerhead Shark has recently been listed as critically endangered on the International Union for Conservation of Nature Red List. Monitoring these endangered species is a challenging procedure since most of the methods used in the process are invasive. Given these circumstances, marine biologists had to look for other options such as filming this species in its natural habitat for further analysis. Posterior inspection of recorded footage helps to monitor the status of the population, but the workload and associated costs are high. Automatic detection systems arise as an essential and innovative solution to this problem. In this sense, we propose an object detection method based on faster regions with convolutional neural networks to detect hammerhead shark species in real-time. The model training used the ResNet50 deep architecture as the feature extractor. After that, it was applied to a real-time tracking scenario to observe the behavior and movement of the hammerhead sharks communities. The obtained average scores of precision (0.82), recall (0.78), and accuracy (0.85) on the experimental image and video datasets highlighted the good performance of the developed hammerhead sharks detector, enabling it as a flexible tool for helping marine biologists in the conservation of this species.
Año de publicación:
2020
Keywords:
- deep learning
- object detection
- hammerhead shark detection and tracking
- ResNet50
- Faster R-CNN
- object tracking
- Real-time detector
Fuente:
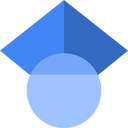
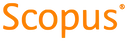
Tipo de documento:
Conference Object
Estado:
Acceso restringido
Áreas de conocimiento:
- Visión por computadora
- Ciencias de la computación
Áreas temáticas:
- Métodos informáticos especiales
- Funcionamiento de bibliotecas y archivos
- Física aplicada