Hand Gesture Recognition Using Leap Motion Controller, Infrared Information, and Deep Learning Framework
Abstract:
Hand gesture recognition (HGR) systems are the current topic, attracting interest in many fields. This broad interest is because people use hand movements to communicate and interact with the physical world. HGR systems are overgrowing, and the reason is that they have applications for different fields of study. Fields can be human-computer interaction (HIC), augmented and virtual reality, robotics, medicine, and video games. Recognizing the frames to correspond to the hand gesture from a frames sequence is essential to developing HIC systems. Thus, this paper presents algorithms to detect the images corresponding to a hand gesture from a frame sequence acquired by the Leap Motion Controller. The frames sequence contains non-gestures images because the movement follows a video pattern in which the initial and final images correspond to the transition of the gesture. Therefore, this paper develops an automatic (AID) and manual (MID) images discriminator. Every algorithm returns a dataset with images corresponding to the hand gesture. To validate the algorithms, we present an HGR model with every algorithm. The models take as input the new dataset and feed an architecture based on convolutional neural networks (CNN). Our models recognize five static gestures: open hand, fist, wave in, wave out and pinch. The results show a classification accuracy of 92.31% with MID and 94.70% with AID.
Año de publicación:
2022
Keywords:
- Leap motion controller
- Hand gesture recognition
- Convolutional neural network
Fuente:
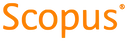
Tipo de documento:
Conference Object
Estado:
Acceso restringido
Áreas de conocimiento:
- Aprendizaje automático
- Ciencias de la computación
Áreas temáticas:
- Métodos informáticos especiales
- Ciencias de la computación