Heuristic Strategies for NFV-Enabled Renewable and Non-Renewable Energy Management in the Future IoT World
Abstract:
The ever-growing energy demand and the CO2 emissions caused by energy production and consumption have become critical concerns worldwide and drive new energy management and consumption schemes. In this regard, energy systems that promote green energy, customer-side participation enabled by the Internet of Things (IoT) technologies, and adaptive consumption mechanisms implemented on advanced communications technologies such as the Network Function Virtualization (NFV) emerge as sustainable and de-carbonized alternatives. On these modern schemes, diverse management algorithmic solutions can be deployed to promote the interaction between generation and consumption sides and optimize the use of available energy either from renewable or non-renewable sources. However, existing literature shows that management solutions considering features such as the dynamic nature of renewable energy generation, prioritization in energy provisioning if needed, and time-shifting capabilities to adapt the workloads to energy availability present a complexity NP-Hard. This condition imposes limits on applicability to a small number of energy demands or time-shifting values. Therefore, faster and less complex adaptive energy management approaches are needed. To meet these requirements, this paper proposes three heuristic strategies: a greedy strategy (GreedyTs), a genetic-algorithm-based solution (GATs), and a dynamic programming approach (DPTs) that, when deployed at the NFV domain, seeks the best possible scheduling of demands that lead to efficient energy utilization. The performance of the algorithmic strategies is validated through extensive simulations in several scenarios, demonstrating improvements in energy consumption and processing of demands. Additionally, simulation results reveal that the heuristic approaches produce high-quality solutions close to the optimal while executing among two and seven orders of magnitude faster and with applicability to scenarios with thousands and hundreds of thousands of energy demands.
Año de publicación:
2021
Keywords:
- Dynamic programming
- greedy algorithm
- Workload scheduling
- Demand Response
- energy management
- Energy efficiency
- Power consumption
- NFV
- RENEWABLE ENERGY
- IOT
- Genetic Algorithm
Fuente:
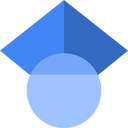
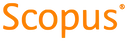
Tipo de documento:
Article
Estado:
Acceso abierto
Áreas de conocimiento:
- Energía
- Energía
- Energía renovable
Áreas temáticas de Dewey:
- Ciencias de la computación
- Economía de la tierra y la energía
- Física aplicada

Objetivos de Desarrollo Sostenible:
- ODS 7: Energía asequible y no contaminante
- ODS 13: Acción por el clima
- ODS 9: Industria, innovación e infraestructura
