Hidden Markov Model based Stochastic Resonance and its Application to Bearing Fault Diagnosis
Abstract:
Rolling bearings are crucial components in rotating machinery and the detection of damage at early stages is pivotal to ensure the life of the machine. Thus, developing accurate methods to identify the presence of faults captured using vibration measurements early on are necessary. Stochastic resonance (SR) is an interesting technique for bearing fault diagnosis, which by adding proper amount of noise to the signal the faulty signal can be extracted. However, traditional SR methods require that the analyst already know the characteristic frequency associated with the fault to be able to extract the corresponding weak signal. This paper overcomes this shortage by adaptively searching the SR system parameters by using Box-Cox sparse measures (BCSM) – based continuous hidden Markov model (CHMM) to determine the characteristic frequency directly from measured data. The method consists of a training phase that uses a simulated outer race fault to determine the optimal CHMM parameters and an application phase where the CHMM is used to determine the characteristic frequency directly from the unprocessed measured signal. The proposed method is verified using three well-known real bearing data sets and is found to be successful in all applications considered here.
Año de publicación:
2022
Keywords:
- Continuous hidden Markov model
- Stochastic resonance
- Parameter Tuning
- Rolling bearing fault diagnosis
- Box-Cox sparse measures
Fuente:
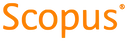
Tipo de documento:
Article
Estado:
Acceso restringido
Áreas de conocimiento:
- Algoritmo
- Aprendizaje automático
Áreas temáticas:
- Ciencias de la computación