Hierarchical Reinforcement Learning for Air Combat At DARPA's AlphaDogfight Trials
Abstract:
Autonomous control in high-dimensional, continuous state spaces is a persistent and important challenge in the fields of robotics and artificial intelligence. Because of high risk and complexity, the adoption of AI for autonomous combat systems has been a long-standing difficulty. In order to address these issues, DARPA's AlphaDogfight Trials (ADT) program sought to vet the feasibility of and increase trust in AI for autonomously piloting an F-16 in simulated air-to-air combat. Our submission to ADT solves the high-dimensional, continuous control problem using a novel hierarchical deep reinforcement learning approach consisting of a high-level policy selector and a set of separately trained low-level policies specialized for excelling in specific regions of the state space. Both levels of the hierarchy are trained using off-policy, maximum entropy methods with expert knowledge integrated through reward shaping. Our approach outperformed human expert pilots and achieved a second-place rank in the ADT championship event. <italic>Impact Statement–</italic> Significant performance milestones in reinforcement learning have been achieved in recent years, with autonomous agents demonstrating super-human performance across a wide variety of tasks. Before these algorithms can be extensively deployed in real-world defense applications, a greater level of trust must first be achieved. ADT was an important step towards developing the trust necessary to operationalize these algorithms, by demonstrating their effectiveness on a foundational yet relevant problem in a high-fidelity simulation environment. Developed for the program, our hierarchical reinforcement learning agent was designed alongside of and competed against active fighter pilots, and ultimately defeated a graduate of the United States Air Force's F-16 Weapons Instructor Course in match play.
Año de publicación:
2022
Keywords:
- Task analysis
- Artificial Intelligence
- hierarchical reinforcement learning
- Atmospheric modeling
- Deep reinforcement learning
- reinforcement learning
- Artificial Intelligence
- Weapons
- entropy
- TRAINING
- Autonomy
- Air combat
Fuente:
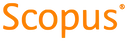
Tipo de documento:
Article
Estado:
Acceso restringido
Áreas de conocimiento:
- Inteligencia artificial
- Simulación por computadora
Áreas temáticas de Dewey:
- Ciencias de la computación