Hierarchical clustering for collaborative filtering recommender systems
Abstract:
Nowadays, the Recommender Systems (RS) that use Collaborative Filtering (CF) are objects of interest and development. CF allows RS to have a scalable filtering, vary metrics to determine the similarity between users and obtain very precise recommendations when using dispersed data. This paper proposes an RS based in Agglomerative Hierarchical Clustering (HAC) for CF. The databases used for the experiments are released and of high dispersion. We used five HAC methods in order to identify which method provides the best results, we also analyzed similarity metrics such as Pearson Correlation (PC) and Jaccard Mean Square Difference (JMSD) versus Euclidean distance. Finally, we evaluated the results of the proposed algorithm through precision, recall and accuracy.
Año de publicación:
2019
Keywords:
- Similarity metrics
- Agglomerative hierarchical clustering
- recommender systems
- COLLABORATIVE FILTERING
Fuente:
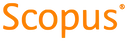
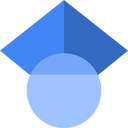
Tipo de documento:
Conference Object
Estado:
Acceso restringido
Áreas de conocimiento:
- Aprendizaje automático
- Ciencias de la computación
- Ciencias de la computación
Áreas temáticas de Dewey:
- Métodos informáticos especiales

Objetivos de Desarrollo Sostenible:
- ODS 9: Industria, innovación e infraestructura
- ODS 17: Alianzas para lograr los objetivos
- ODS 8: Trabajo decente y crecimiento económico
