Hierarchical genetic algorithms for fuzzy inference system optimization applied to response integration for pattern recognition
Abstract:
In this paper, a new method for fuzzy inference system optimization is proposed. The optimization consists in find the optimal parameters of fuzzy inference system used to combine the responses of modular neural networks using a hierarchical genetic algorithm. The optimized parameters are: type of fuzzy logic (type-1 and interval type-2), type of system (Mamdani or Sugeno), type of membership functions, number of membership functions in each variable (inputs and output), their parameters and the consequents of the fuzzy rules. Four benchmark databases are used to test the proposed method where, each database is a different biometric measure (face, iris, ear and voice) and each database is learned by a modular neural network. The main objective of the fuzzy inference system is to combine the different responses of the modular neural network and achieve final good results even when one (o more …
Año de publicación:
2014
Keywords:
Fuente:
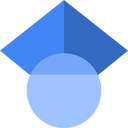
Tipo de documento:
Other
Estado:
Acceso abierto
Áreas de conocimiento:
- Aprendizaje automático
- Optimización matemática
Áreas temáticas:
- Métodos informáticos especiales