Human Action Recognition in Video Sequence using Logistic Regression by Features Fusion Approach based on CNN Features
Abstract:
Human Action recognition (HAR) gains too much attention due to its wide range of real world applications, such as video surveillance, robotics and computer vision. In video surveillance systems security cameras are placed to monitor activities and motion, generate alerts in undesirable situations. Due to such importance of video surveillance in daily life, HAR becomes the primary and key factor of video surveillance systems. Many researchers worked on human action recognition but HAR still a challenging problem, due to large variation among human to human and human actions in daily life, which make human recognition very challenging and makes surveillance system difficult to outperform. In this article a novel method is proposed by features fusion of pre-trained convolution neural network (CNN) features. Initially pre-trained CNN VGG 19 weights are exploited to extract fully connected 7th layer (FC7) of the selected dataset, subsequently pre-trained fully connected 8th layer features (FC8) extracted by employing pre-trained weights of the same neural network. However the resultant feature fused vector further optimized by employing two statistical features selection techniques, chi-square test and mutual information to select best features among them to reduced redundancy and increase performance accuracy of human action, a threshold value used for selecting best features. Furthermore the best features are fused, then grid search with 10 fold cross validation is applied for tuning hyper parameter to select best k fold and the resulting best parameter are feed to Logistic regression (LR) classifier for recognition. The proposed technique used You Tube 11 action dataset and achieved 98.49% accuracy. Lastly the proposed method compares with the existing state of the art methods which show dominance performance.
Año de publicación:
2021
Keywords:
- features fusion
- Human Action Recognition
- convolution neural network
- logistic regression
- deep learning
Fuente:
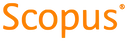
Tipo de documento:
Article
Estado:
Acceso abierto
Áreas de conocimiento:
- Aprendizaje automático
- Ciencias de la computación
Áreas temáticas:
- Ciencias de la computación