Human-Assisted Signature Recognition Based on Comparative Attributes
Abstract:
This work analyzes the performance of comparative attributes labeled by humans for handwritten semi-automatic signature recognition. Despite the large deployment of automatic sys-tems, humans have still an important role in many tasks relat-ed to handwritten signature recording and verification. How humans can help to improve these processes is a primary aim of different research lines. Comparative attributes try to ex-ploit the abilities of humans to extract discriminant infor-mation of the signatures. Instead of absolute attributes (e.g. is this stroke vertical?), the comparative attributes offer richer responses (e.g. how vertical is this stroke?). In this work we present a new semi-automatic signature labeling interface in-spired by Forensic Document Examiners (FDE). Fifteen com-parative attributes have been labeled by 21 laymen, where each one carries out the labeling of 28 signatures from 130 users of the publicly available corpus BiosecurID database. Through the manual labeling, a new Bio-HSL (Biometric-Handwritten Signatures Labelling) database is generated, which contains 4,968,600 signature attributes. The results show that compara-tive attributes outperform absolute attributes for semi-automatic signature recognition with Equal Error Rates ranging from 5.5% for random comparisons to 21.2% for simulated forgeries.
Año de publicación:
2018
Keywords:
- Signature recognition
- Biometrics
- Forensics
- Human in the loop
Fuente:
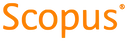
Tipo de documento:
Conference Object
Estado:
Acceso restringido
Áreas de conocimiento:
- Aprendizaje automático
- Ciencias de la computación
Áreas temáticas de Dewey:

Objetivos de Desarrollo Sostenible:
- ODS 9: Industria, innovación e infraestructura
- ODS 16: Paz, justicia e instituciones sólidas
- ODS 8: Trabajo decente y crecimiento económico
