Human-like Object Grasping and Relocation for an Anthropomorphic Robotic Hand with Natural Hand Pose Priors in Deep Reinforcement Learning
Abstract:
Anthropomorphic manipulators such as robotic hands (i.e., agent) can be used to perform complex object grasping tasks similar to human hands. However, teaching an agent to perform human-like grasping of objects is still a challenge in the robotics community. In this work, natural hand poses (i.e., priors) according to the shape of objects are used in training an agent via Deep Reinforcement Learning (DRL). To validate the effect of humanlike grasping priors, the agent is trained to perform object grasping and relocation tasks including a single apple, milk box, and can. The results show that the trained agent with human-like hand pose priors in DRL is able to grasp the objects more naturally and performs object relocation with significantly improved success rate against the trained agent with only DRL.
Año de publicación:
2019
Keywords:
- Deep Reinforcement Learning.
- Anthropomorphic Hand Manipulation
- Natural Hand Pose Prior
- Human-Like Object Grasping
Fuente:
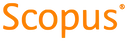
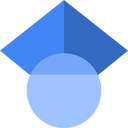