Hybrid Deep Learning Architecture Approach for Photovoltaic Power Plant Output Pbkp_rediction
Abstract:
Photovoltaic Power is an interesting type of renewable energy, but the intermittency of solar energy resources makes its pbkp_rediction an challenging task. This article presents the performance of a Hybrid Convolutional - Long short term memory network (CNN-LSTM) architecture in the pbkp_rediction of photovoltaic generation. The combination was deemed important, as it can integrate the advantages of both deep learning methodologies: the spatial feature extraction and speed of CNN and the temporal analysis of the LSTM. The developed 4 layer Hybrid CNN-LSTM (HCL) model was applied on a real-world data collection for Photovoltaic Power pbkp_rediction on which Group Least Square Support Vector Machines (GLSSVM) reported the lowest error in the current state of the art. Alongside the PV output, 4 other pbkp_redictors are included in the models. The main result obtained from the evaluation metrics reveals that the proposed HCL provides better pbkp_rediction than the GLSSVM model since the MSE and MAE errors of HCL are significantly lower than the same errors of the GLSSVM. So, the proposed Hybrid CNN-LSTM architecture is a promising approach for increasing the accuracy in Photovoltaic Power Pbkp_rediction.
Año de publicación:
2022
Keywords:
- Photovoltaic
- pbkp_rediction
- cnn
- deep learning
- LSTM
Fuente:
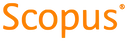
Tipo de documento:
Conference Object
Estado:
Acceso restringido
Áreas de conocimiento:
- Fotovoltaica
- Inteligencia artificial
- Energía renovable
Áreas temáticas de Dewey:
- Física aplicada
- Métodos informáticos especiales