Hybrid models based on singular values and autoregressive methods for multistep ahead forecasting of traffic accidents
Abstract:
The traffic accidents occurrence urges the intervention of researchers and society; the human losses and material damage could be abated with scientific studies focused on supporting prevention plans. In this paper prediction strategies based on singular values and autoregressive models are evaluated for multistep ahead traffic accidents forecasting. Three time series of injured people in traffic accidents collected in Santiago de Chile from 2000:1 to 2014:12 were used, which were previously classified by causes related to the behavior of drivers, passengers, or pedestrians and causes not related to the behavior as road deficiencies, mechanical failures, and undetermined causes. A simplified form of Singular Spectrum Analysis (SSA), combined with the autoregressive linear (AR) method, and a conventional Artificial Neural Network (ANN) are proposed. Additionally, equivalent models that combine Hankel Singular Value Decomposition (HSVD), AR, and ANN are evaluated. The comparative analysis shows that the hybrid models SSA-AR and SSA-ANN reach the highest accuracy with an average MAPE of 1.5% and 1.9%, respectively, from 1- to 14-step ahead prediction. However, it was discovered that HSVD-AR shows a higher accuracy in the farthest horizons, from 12- to 14-step ahead prediction, which reaches an average MAPE of 2.2%.
Año de publicación:
2016
Keywords:
Fuente:
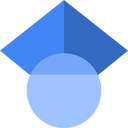
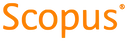
Tipo de documento:
Article
Estado:
Acceso abierto
Áreas de conocimiento:
- Transporte
- Análisis de datos
- Estadísticas
Áreas temáticas de Dewey:
- Ciencias de la computación
- Lingüística
- Otras ramas de la ingeniería

Objetivos de Desarrollo Sostenible:
- ODS 3: Salud y bienestar
- ODS 11: Ciudades y comunidades sostenibles
- ODS 9: Industria, innovación e infraestructura
