I-vector analysis for Gait-based Person Identification using smartphone inertial signals
Abstract:
This paper describes and evaluates an i-vector based approach for Gait-based Person Identification (GPI) using inertial signals from a smartphone. This approach includes two variability compensation strategies (Linear Discrimination Analysis (LDA) and Probabilistic LDA) for dealing with the gait variability due to different recording sessions or different activities carried out by the user. This study uses a public available dataset that includes recordings from 30 users performing three different activities: walking, walking-upstairs and walking-downstairs. The i-vector approach is compared to a Gaussian Mixture Model-Universal Background Model (GMM-UBM) system, providing significant performance improvements when incorporating the PLDA compensation strategy: the best result reports a User Recognition Error Rate (URER) of 17.7%, an Equal Error Rate (EER) of 6.1% and an F1-score of 82.7% with 30 enrolled users. For less than six enrolled users, the URER error decreases to 5%.
Año de publicación:
2017
Keywords:
- Person identification
- gait recognition
- I-vector analysis
- PLDA compensation
- Smartphone inertial signals
Fuente:
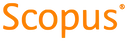
Tipo de documento:
Article
Estado:
Acceso restringido
Áreas de conocimiento:
- Aprendizaje automático
- Ciencias de la computación
Áreas temáticas de Dewey:
- Ciencias de la computación

Objetivos de Desarrollo Sostenible:
- ODS 3: Salud y bienestar
- ODS 17: Alianzas para lograr los objetivos
- ODS 9: Industria, innovación e infraestructura
