Identification of continuous-time systems utilising Kautz basis functions from sampled-data
Abstract:
In this paper we address the problem of identifying a continuous-time deterministic system utilising sampled-data with instantaneous sampling. We develop an identification algorithm based on Maximum Likelihood. The exact discrete-time model is obtained for two cases: i) known continuous-time model structure and ii) using Kautz basis functions to approximate the continuous-time transfer function. The contribution of this paper is threefold: i) we show that, in general, the discretisation of continuous-time deterministic systems leads to several local optima in the likelihood function, phenomenon termed as aliasing, ii) we discretise Kautz basis functions and obtain a recursive algorithm for constructing their equivalent discrete-time transfer functions, and iii) we show that the utilisation of Kautz basis functions to approximate the true continuous-time deterministic system results in convex log-likelihood functions. We illustrate the benefits of our proposal via numerical examples.
Año de publicación:
2020
Keywords:
- system identification
- Kautz basis functions
- Discrete-time model
- continuous-time model
- Maximum likelihood
Fuente:
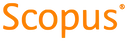
Tipo de documento:
Conference Object
Estado:
Acceso abierto
Áreas de conocimiento:
- Sistema de control
- Teoría de control
Áreas temáticas:
- Física aplicada