Identifying the presence and severity of dementia by applying interpretable machine learning techniques on structured clinical records
Abstract:
Background: Dementia develops as cognitive abilities deteriorate, and early detection is critical for effective preventive interventions. However, mainstream diagnostic tests and screening tools, such as CAMCOG and MMSE, often fail to detect dementia accurately. Various graph-based or feature-dependent pbkp_rediction and progression models have been proposed. Whenever these models exploit information in the patients’ Electronic Medical Records, they represent promising options to identify the presence and severity of dementia more precisely. Methods: The methods presented in this paper aim to address two problems related to dementia: (a) Basic diagnosis: identifying the presence of dementia in individuals, and (b) Severity diagnosis: pbkp_redicting the presence of dementia, as well as the severity of the disease. We formulate these two tasks as classification problems and address them using machine learning models based on random forests and decision tree, analysing structured clinical data from an elderly population cohort. We perform a hybrid data curation strategy in which a dementia expert is involved to verify that curation decisions are meaningful. We then employ the machine learning algorithms that classify individual episodes into a specific dementia class. Decision trees are also used for enhancing the explainability of decisions made by pbkp_rediction models, allowing medical experts to identify the most crucial patient features and their threshold values for the classification of dementia. Results: Our experiment results prove that baseline arithmetic or cognitive tests, along with demographic features, can pbkp_redict dementia and its severity with high accuracy. In specific, our pbkp_rediction models have reached an average f1-score of 0.93 and 0.81 for problems (a) and (b), respectively. Moreover, the decision trees produced for the two issues empower the interpretability of the pbkp_rediction models. Conclusions: This study proves that there can be an accurate estimation of the existence and severity of dementia disease by analysing various electronic medical record features and cognitive tests from the episodes of the elderly population. Moreover, a set of decision rules may comprise the building blocks for an efficient patient classification. Relevant clinical and screening test features (e.g. simple arithmetic or animal fluency tasks) represent precise pbkp_redictors without calculating the scores of mainstream cognitive tests such as MMSE and CAMCOG. Such pbkp_redictive model can identify not only meaningful features, but also justifications of classification. As a result, the pbkp_redictive power of machine learning models over curated clinical data is proved, paving the path for a more accurate diagnosis of dementia.
Año de publicación:
2022
Keywords:
- dementia
- Mini mental score
- CAMCOG
- LIME
- data science
- Machine learning
Fuente:
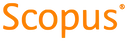
Tipo de documento:
Article
Estado:
Acceso abierto
Áreas de conocimiento:
- Aprendizaje automático
- Imágenes médicas
Áreas temáticas de Dewey:
- Medicina y salud
- Enfermedades
- Ciencias de la computación