Image-based model pbkp_redictive control via dynamic mode decomposition
Abstract:
We present a data-driven model pbkp_redictive control (MPC) framework for systems with high state–space dimensionality. This work is motivated by the need to exploit sensor data that appears in the form of images (e.g., 2D or 3D spatial fields captured by thermal cameras). We propose to use dynamic mode decomposition (DMD) to directly build a low-dimensional model from image data and we use such model to obtain a tractable MPC controller. We demonstrate the scalability of this approach (which we call DMD-MPC) by using a 2D thermal diffusion system. Here, we assume that the evolution of the thermal field is captured by 50 x 50 pixel images, which results in a 2500-dimensional state–space. We show that the dynamics of this high-dimensional space can be accurately captured by using a 40-dimensional DMD model and we show that the field can be manipulated satisfactorily by using an MPC controller that embeds the low-dimensional DMD model. We also show that the DMD-MPC controller outperforms a standard MPC controller that uses data collected from a finite set of spatial locations (proxy locations) to manipulate the high-dimensional thermal field. This illustrates the value of information embedded in image data.
Año de publicación:
2021
Keywords:
- Data-driven
- Dynamic mode decomposition
- Model Pbkp_redictive Control
- Image data
- Model reduction
Fuente:
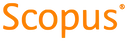
Tipo de documento:
Article
Estado:
Acceso restringido
Áreas de conocimiento:
- Sistema de control
- Control óptimo
Áreas temáticas:
- Métodos informáticos especiales