Improved effort estimation of heterogeneous ensembles using filter feature selection
Abstract:
Estimating the amount of effort required to develop a new software system remains the main activity in software project management. Thus, providing an accurate estimate is essential to adequately manage the software lifecycle. For that purpose, many paradigms have been proposed in the literature, among them Ensemble Effort Estimation (EEE). EEE consists of pbkp_redicting the effort of the new project using more than one single pbkp_redictor. This paper aims at improving the pbkp_rediction accuracy of heterogeneous ensembles whose members use filter feature selection. Three groups of ensembles were constructed and evaluated: ensembles without feature selection, ensembles with one filter, and ensembles with different filters. The overall results suggest that the use of different filters lead to generate more accurate heterogeneous ensembles, and that the ensembles whose members use one filter were the worst ones.
Año de publicación:
2019
Keywords:
- ensemble
- Machine learning
- feature selection
- filter
- Software development effort estimation
Fuente:
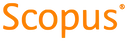
Tipo de documento:
Conference Object
Estado:
Acceso restringido
Áreas de conocimiento:
- Aprendizaje automático
- Software
- Ciencias de la computación
Áreas temáticas:
- Programación informática, programas, datos, seguridad
- Métodos informáticos especiales
- Funcionamiento de bibliotecas y archivos