Improving Classification Models Using the Frequency of Terms and a Percentage Relation Approach Between Classes for Emergency Calls
Abstract:
The analysis of informal texts is essential in text mining applications, such as the classification ones. The division of classes into informal and non-structured text is necessary because it is possible to extract knowledge for each category. The problem emerges in languages because those have high dimensionality. Therefore, the complexity should be reduced by applying techniques that include dimensionality decrease. Based on these arguments, a technique that is pruning the words in medium and long texts was applied through its frequency in all documents, and frequency in each class. This study aims to determine irrelevant and relevant terms that proper fit for each category and to improve the classification performance. This study, including a four-step methodology: i) data preprocessing, ii) analyzing term frequency, iii) reduction language dimensionality, and iv) classification model structuring. For this, a series of experiments were proposed to determine the word frequency threshold, with a decisive index that establishes the words to be eliminated or conserved. The experimentation showed that the application of the word pruning approach significantly improves the performance of the binary classification according to its evaluation measures.
Año de publicación:
2021
Keywords:
- bag-of-words
- TEXT MINING
- Emergency calls
- classification
- pruning
Fuente:
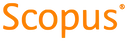
Tipo de documento:
Conference Object
Estado:
Acceso restringido
Áreas de conocimiento:
- Aprendizaje automático
- Ciencias de la computación
Áreas temáticas:
- Ciencias de la computación