Improving Classification Using Topic Correlation and Expectation Propagation
Abstract:
Probabilistic topic models are broadly used to infer meaningful patterns of words over a mixture of latent topics that are commonly used for statistical analyses or as a proxy for supervised tasks. However, models such as Latent Dirichlet Allocation (LDA) assume independence between topic proportions due to the nature of the Dirichlet distribution; this effect is captured with other distributions such as the logistic normal distribution, resulting in a complex model. In this paper, we develop a probabilistic topic model using the generalized Dirichlet distribution (LGDA) in order to capture topic correlation while maintaining conjugacy. We make use of Expectation Propagation to approximate the posterior, resulting in a model that achieves more accurate inferences compared to variational inference. We evaluate the convergence of EP compared with the classical LDA by comparing the approximation to the marginal distribution. We show the obtained topics by LGDA and evaluate its pbkp_redictive performance in two text classification tasks, outperforming the vanilla LDA.
Año de publicación:
2020
Keywords:
- Topic classification
- TOPIC MODELLING
- Expectation propagation
Fuente:
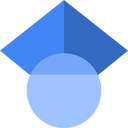
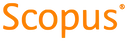
Tipo de documento:
Conference Object
Estado:
Acceso restringido
Áreas de conocimiento:
- Aprendizaje automático
- Algoritmo
Áreas temáticas:
- Funcionamiento de bibliotecas y archivos