Improving LMI controllers for discrete nonlinear systems using policy iteration
Abstract:
This paper presents a method to improve the conservative (shape-independent) controllers from guaranteed-cost LMIs using Q-learning policy iteration setups which learn optimal controllers from data. In this context, the proposed approach uses an initial parametrization based on the LMI solution and the learning process includes parameters related to the nonlinearity of the system (and its gradient). An approximation of the Q-function using polynomials of the membership functions in Takagi-Sugeno models is obtained using a policy iteration algorithm. The resulting controller is shape-dependent, that is, uses the knowledge of membership functions, their gradients and data to improve well-known LMI solutions.
Año de publicación:
2017
Keywords:
- Q-Learning
- LMI
- policy iteration (pI)
- Takagi-Sugeno
- reinforcement learning
Fuente:
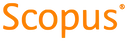
Tipo de documento:
Conference Object
Estado:
Acceso restringido
Áreas de conocimiento:
- Algoritmo
- Teoría de control
Áreas temáticas:
- Ciencias de la computación