Improving aerosol optical depth retrievals from Himawari-8 with ensemble learning enhancement: Validation over Asia
Abstract:
The Advanced Himawari Imager (AHI) onboard the Himawari-8 spacecraft from the Japan Meteorological Agency (JMA) enables retrieval of aerosol optical depth (AOD) with high spatio-temporal resolution. This work demonstrates that the latest version of Level-3 AHI AOD product is affected by an overall underestimation against ground measurements from 98 sunphotometer sites over Asia, with site-average R2 and mean bias error (MBE) of 0.54 and − 0.10, respectively. The MBE varies from −0.23 to 0.03 over land, depending on site, and averages 0.06 over water. To correct these errors, an XGBoost model has been developed based on the AHI AOD, as well as meteorological quantities and geographic information from the ERA5 ECMWF reanalysis, a digital elevation model (DEM), and ground-based AOD measurements obtained in 2018. After application of this correction algorithm, the testing dataset indicates that R2 and the fraction of data included within the expected error (EE) envelope (±15%) are 0.86 and 74.7%, respectively, thus representing substantial improvement compared to the corresponding uncorrected results of 0.59 and 42.2%. The optimal XGBoost also demonstrates a good generalization ability because 69.5% of the corrected AOD falls within the EE envelope when applying the model to AHI data from 2019. The model's Feature Importance analysis shows that, besides the raw AHI AOD, geographic coordinates, surface albedo, and atmospheric water vapor contribute significantly to the correction model. The AOD difference map (corrected AOD minus raw AHI AOD) reveals that the XGBoost model improves AOD values considerably for land areas by 0.14, 0.10, 0.09, and 0.11 for MAM, JJA, SON and DJF, respectively.
Año de publicación:
2023
Keywords:
- Correction algorithm
- Himawari-8
- AOD
- AERONET
- CARSNET
- XGBoost
Fuente:
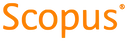
Tipo de documento:
Article
Estado:
Acceso restringido
Áreas de conocimiento:
- Ciencia ambiental
- Ciencia atmosférica
- Aprendizaje automático
Áreas temáticas:
- Geología, hidrología, meteorología
- Dirección general
- Métodos informáticos especiales