Improving core topics discovery in semantic markup literature: A combined approach
Abstract:
This research configures a corpus of articles related to the aspects being investigated in Semantic Markup, knowledge domain that has evolved and expanded over the last decade and conduct a manual process to identify the Topics being addressed. Then, it is used LDA, an unsupervised probabilistic topic model, and other tools, for automatically recognize the topics of interest within this corpus; this aims to interpret, validate and complement the results manually obtained. The results let us argue that using combined techniques contribute to improving the human expert analysis, and it is helpfully for the discovery of core topics in Semantic Markup Literature.
Año de publicación:
2020
Keywords:
- Topic Models
- Systematic mapping
- Latent Dirichlet Allocation
- Embedded semantic markup
Fuente:
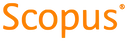
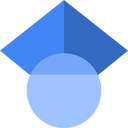
Tipo de documento:
Conference Object
Estado:
Acceso restringido
Áreas de conocimiento:
- Web Semántica
- Ciencias de la computación
Áreas temáticas:
- Funcionamiento de bibliotecas y archivos