Improving feature extraction in the automatic classification of seismic events. Application to Colima and Arenal volcanoes
Abstract:
Monitoring of precursory seismicity in volcanoes is the most reliable and widely used technique in volcano monitoring. Since a visual inspection by human operators is a tedious task in a non-stop monitoring process, Hidden Markov Models have been previously proposed to automatically classify the different types of volcano-seismic events. Mel Frequency Cepstral Coefficients were successfully used as feature vector in this continuous classification system. In this paper seven novel features to be included in the MFCC feature vector are proposed. A very elementary GMM-based classifier has been implemented in order to assess the efficiency of the proposed parameters. Results using hundreds of events recorded from stations situated at Colima (Mexico) and Arenal (Costa Rica) volcanoes show that the proposed features improve the recognition accuracy and therefore they may be relevant in continuous volcano-seismic event automatic classification. ©2009 IEEE.
Año de publicación:
2009
Keywords:
- Hidden markov models
- Volcano-seismic events
- classification
- MFCC
- Feature vector
Fuente:
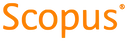
Tipo de documento:
Conference Object
Estado:
Acceso restringido
Áreas de conocimiento:
- Sismología
- Sismología
- Aprendizaje automático
Áreas temáticas de Dewey:
- Ciencias de la tierra
- Química y ciencias afines
- Ingeniería y operaciones afines

Objetivos de Desarrollo Sostenible:
- ODS 9: Industria, innovación e infraestructura
- ODS 13: Acción por el clima
- ODS 17: Alianzas para lograr los objetivos
