Improving hourly precipitation estimates for flash flood modeling in data-scarce andean-amazon basins: An integrative framework based on machine learning and multiple remotely sensed data
Abstract:
Accurate estimation of spatiotemporal precipitation dynamics is crucial for flash flood forecasting; however, it is still a challenge in Andean-Amazon sub-basins due to the lack of suitable rain gauge networks. This study proposes a framework to improve hourly precipitation estimates by integrating multiple satellite-based precipitation and soil-moisture products using random forest modeling and bias correction techniques. The proposed framework is also used to force the GR4H model in three Andean-Amazon sub-basins that suffer frequent flash flood events: upper Napo River Basin (NRB), Jatunyacu River Basin (JRB), and Tena River Basin (TRB). Overall, precipitation estimates derived from the framework (BC-RFP) showed a high ability to reproduce the intensity, distribution, and occurrence of hourly events. In fact, the BC-RFP model improved the detection ability between 43% and 88%, reducing the estimation error between 72% and 93%, compared to the original satellite-based precipitation products (i.e., IMERG-E/L, GSMAP, and PERSIANN). Likewise, simulations of flash flood events by coupling the GR4H model with BC-RFP presented satisfactory performances (KGE* between 0.56 and 0.94). The BC-RFP model not only contributes to the implementation of future flood forecast systems but also provides relevant insights to several water-related research fields and hence to integrated water resources management of the Andean-Amazon region.
Año de publicación:
2021
Keywords:
- GR4H model
- PERSIANN
- Complex topography areas
- Upper Napo River Basin
- SMAP
- IMERG
- GSMAP
Fuente:
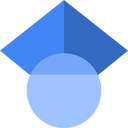
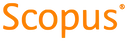
Tipo de documento:
Article
Estado:
Acceso abierto
Áreas de conocimiento:
- Hidrología
- Aprendizaje automático
- Ciencia ambiental
Áreas temáticas:
- Física aplicada
- Métodos informáticos especiales
- Ciencias de la tierra